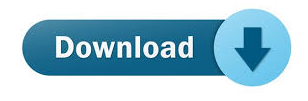

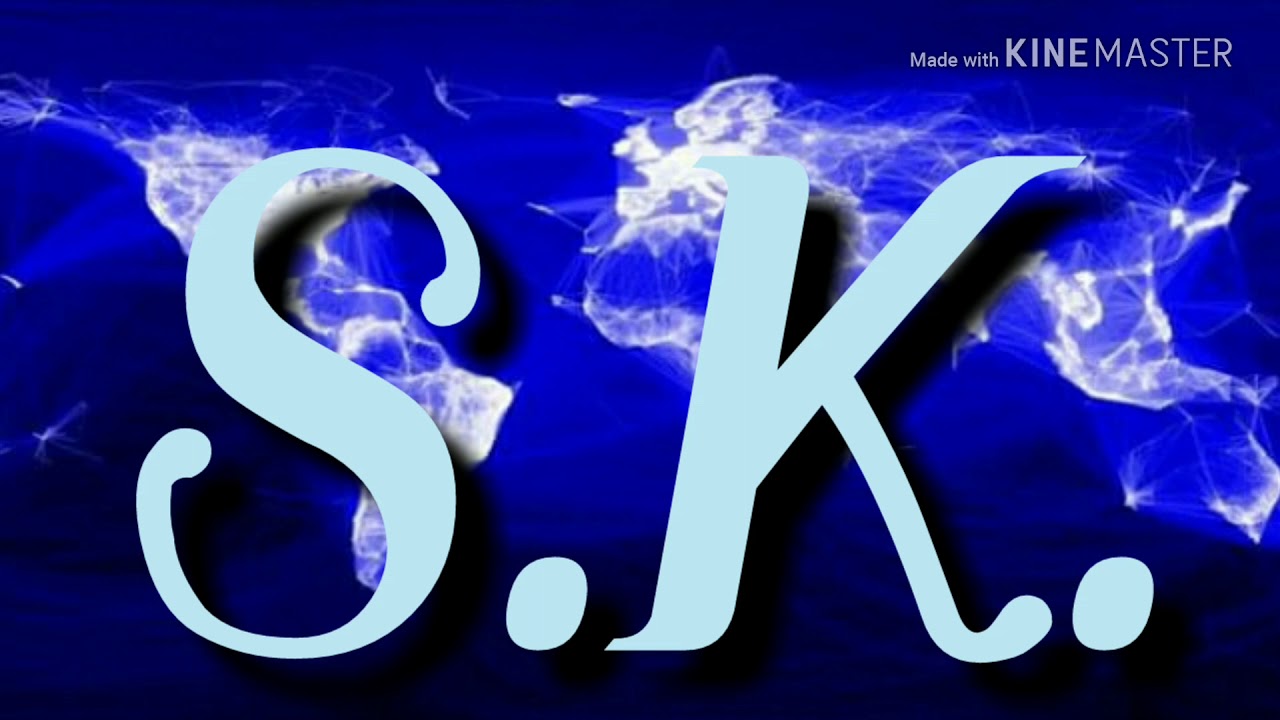
Further, we implement a nonlinear support vector machine with optimized hyperparameters for training and predicting the features to generate the scores. Then, the proposed approach extracts several features from captured behavioral data and selects the best set of features through a filter-based feature selection technique. The proposed approach captures the touch and motion-based behavioral biometrics through the touchscreen and inertial sensors of the device. This article introduces a sensor-based continuous user authentication approach on the smartphone through multi-modal behavioral biometrics and a machine learning model to tackle the aforementioned issues. Many entry-point authentication methods have failed to offer security due to insider or side-channel attacks. This has fueled the importance of entry-point authentication methods on smartphones. Thus, the security of stored data on smartphones has become a significant concern. With the rapid development of hardware and software technology, many end-users have often stored their data on smartphones through several in-built sensors.
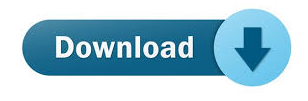